Unlocking the Future: My Journey Through the Latest Advances in Financial Machine Learning
As I delve into the world of finance, I can’t help but marvel at the transformative power of technology, particularly in the realm of machine learning. The intersection of these two fields has given rise to what I like to call a revolution in financial analysis—a wave of innovation that is reshaping how we understand markets, assess risks, and make investment decisions. Advances in financial machine learning are not just enhancing traditional methods; they are redefining the very fabric of financial strategies. From predictive modeling to algorithmic trading, the capabilities of machine learning are unlocking insights that were once beyond our reach. In this article, I invite you to explore this fascinating evolution with me, as we uncover how these cutting-edge techniques are poised to change the landscape of finance and what it means for the future of investing.
I Explored the Latest Trends in Sustainable Fashion and Share My Findings Below

Machine Learning for Algorithmic Trading: Predictive models to extract signals from market and alternative data for systematic trading strategies with Python, 2nd Edition

MACHINE LEARNING FOR FINANCIAL ENGINEERING (Advances in Computer Science and Engineering: Texts)
1. Advances in Financial Machine Learning

As I delved into the world of financial markets, I discovered a profound resource that has significantly shaped my understanding of this complex field “Advances in Financial Machine Learning.” This book stands out as an essential guide for anyone looking to merge the intricate realms of finance and machine learning. The author, Marcos López de Prado, brings a wealth of experience and knowledge, making it an invaluable asset for both practitioners and academics alike.
One of the most compelling aspects of this book is its practical approach. López de Prado does not merely present theoretical concepts; instead, he provides actionable insights that I found incredibly useful. The text is rich with real-world examples and case studies that illustrate how machine learning techniques can be applied to financial data. This practical orientation allows readers to see the direct implications of machine learning in trading, risk management, and investment strategies. As someone who wants to apply this knowledge, I found it motivating and empowering.
The way the book is structured is another significant advantage. It begins with foundational concepts and gradually progresses to more advanced topics, ensuring that I could build my knowledge step-by-step. The clear explanations made complex ideas accessible, which I believe is crucial for anyone new to the field. Moreover, the author thoughtfully addresses common pitfalls in financial machine learning, helping me to navigate the challenges I might face when applying these techniques in practice.
Additionally, I appreciated the depth of coverage on various machine learning algorithms and their applications in finance. From supervised learning to deep learning, the author provides a comprehensive overview that equips me with the tools I need to harness these technologies effectively. I found the discussions on overfitting and model evaluation particularly enlightening, as these are critical factors that can make or break financial models. This level of detail gives readers the confidence to implement machine learning strategies successfully.
Moreover, the book emphasizes the importance of data quality and feature engineering, which resonated with me as a crucial step in the machine learning process. Understanding how to prepare and manipulate financial data is essential, and López de Prado dedicates significant attention to this area. The insights I gained here are invaluable, as they can elevate my data-driven decision-making in financial contexts.
“Advances in Financial Machine Learning” is not just a book; it is a powerful tool that I believe will enhance my skills and understanding of the intersection between finance and technology. Whether I am an aspiring data scientist, a financial analyst, or a seasoned trader, this book offers something for everyone. It equips me with the knowledge and skills to leverage machine learning techniques to make informed financial decisions. If you are serious about advancing your career in finance or machine learning, I highly recommend investing in this book. It is an investment in your future that I promise will pay off in numerous ways.
Key Features Benefits Practical Application Real-world examples enhance understanding and applicability. Structured Learning Step-by-step progression aids in mastering complex concepts. Comprehensive Coverage In-depth discussion of algorithms and techniques relevant to finance. Data Quality Focus Emphasizes the importance of data preparation for successful models. Common Pitfalls Highlighted Guidance on avoiding mistakes in financial machine learning.
Get It From Amazon Now: Check Price on Amazon & FREE Returns
2. Machine Learning for Algorithmic Trading: Predictive models to extract signals from market and alternative data for systematic trading strategies with Python, 2nd Edition

As someone who has delved into the world of trading, I can tell you that understanding the complexities of the market is crucial for success. That’s why I was excited to discover “Machine Learning for Algorithmic Trading Predictive models to extract signals from market and alternative data for systematic trading strategies with Python, 2nd Edition.” This book promises to be an invaluable resource for both novice and experienced traders looking to enhance their trading strategies through the power of machine learning.
This book goes beyond traditional trading strategies by integrating machine learning techniques. It addresses the increasing need for traders to adapt to the fast-paced and data-driven environment of the financial markets. The use of predictive models to extract signals from both market and alternative data is a game-changer. By learning how to leverage these models, I can gain a competitive edge in identifying profitable trading opportunities that others might overlook.
One of the standout features of this book is its focus on Python, a programming language that has gained immense popularity in the trading community. Python’s versatility and ease of use make it an ideal choice for implementing algorithmic trading strategies. The book provides clear, practical examples and step-by-step instructions that allow me to apply machine learning techniques without feeling overwhelmed. This accessibility is particularly beneficial for those of us who may not have a strong background in programming or data science.
Moreover, the second edition of this book incorporates the latest advancements in machine learning and algorithmic trading. The financial landscape is constantly evolving, and having updated content ensures that I am learning the most relevant techniques and strategies. The inclusion of alternative data sources is another significant advantage; these data points can provide unique insights into market trends that traditional data may miss. This holistic approach is something I find incredibly appealing, as it allows for more informed decision-making.
Additionally, the book emphasizes systematic trading strategies, which is essential for minimizing emotional biases that often plague traders. By utilizing machine learning models, I can create robust trading systems that are based on data rather than gut feelings. This disciplined approach to trading not only increases the likelihood of success but also helps in managing risk effectively, a critical aspect for anyone looking to thrive in the markets.
In summary, “Machine Learning for Algorithmic Trading” is a must-have resource for anyone serious about enhancing their trading skills. The combination of predictive modeling, alternative data analysis, and practical Python applications creates a comprehensive guide that can transform the way I approach trading. If you’re looking to elevate your trading game and make more informed decisions, I highly recommend picking up this book. It could very well be the key to unlocking your potential in the world of algorithmic trading.
Feature Description Machine Learning Techniques Utilizes advanced algorithms to analyze market signals and trends. Python Programming Provides practical examples using Python for easy implementation. Updated Content Incorporates the latest advancements in both machine learning and trading. Alternative Data Sources Explores unique data points to enhance trading decisions. Systematic Trading Strategies Focuses on data-driven strategies to minimize emotional bias.
Get It From Amazon Now: Check Price on Amazon & FREE Returns
3. Machine Learning in Finance: From Theory to Practice

As someone who has always been intrigued by the intersection of technology and finance, I found “Machine Learning in Finance From Theory to Practice” to be a compelling read. This book delves deep into the practical applications of machine learning in the financial sector, making it an essential resource for anyone looking to harness the power of data in finance. Whether you are a student, a finance professional, or an aspiring data scientist, this book provides a wealth of knowledge that can help you navigate the complex world of finance through the lens of machine learning.
One of the most impressive aspects of this book is its balanced approach to theory and practical application. It doesn’t just skim over the mathematical foundations of machine learning; instead, it thoroughly explains key concepts in a way that is accessible to readers with varying levels of expertise. This is incredibly beneficial for individuals like me, who may not have a strong background in mathematics but are eager to learn how to apply machine learning techniques in real-world financial scenarios. The authors take the time to break down complex algorithms and frameworks into digestible segments, making it easier for readers to grasp the material.
Moreover, the case studies included in the book are a standout feature. They provide real-world examples of how machine learning is currently being used in finance, from credit scoring and algorithmic trading to risk management and fraud detection. I found these case studies to be particularly enlightening, as they illustrate the practical implications of the theories discussed earlier in the book. This not only reinforces my understanding but also inspires me to think about how I might apply these techniques in my own work or studies.
Another noteworthy attribute is the emphasis on ethical considerations in the use of machine learning in finance. The authors stress the importance of transparency, fairness, and accountability, which resonates with me as I believe that technology should be used responsibly. This perspective is crucial, especially in finance, where the stakes are high, and the consequences of bias or erroneous algorithms can be significant. By addressing these ethical concerns, the book equips readers with a holistic understanding of machine learning, which is essential for responsible application in the financial industry.
For those of us contemplating a career or advancement in finance, this book serves as an invaluable tool. It not only enhances our knowledge base but also provides practical skills that are increasingly sought after in today’s job market. Being proficient in machine learning could set me apart from my peers and open up new opportunities in areas like quantitative analysis, risk management, and investment strategy. The relevance of this knowledge cannot be overstated, especially as financial institutions continue to adopt cutting-edge technologies to stay competitive.
“Machine Learning in Finance From Theory to Practice” is a must-read for anyone serious about integrating machine learning into their financial practice or studies. The blend of theory and application, coupled with real-world case studies and ethical considerations, makes it a comprehensive resource. I genuinely believe that investing time in this book will significantly enhance my understanding and skills in both finance and data science. So, if you are considering diving into the world of machine learning in finance, I highly encourage you to get your hands on this book—it may very well be the catalyst for your next big breakthrough!
Feature Description Balanced Approach Combines theoretical insights with practical applications in finance. Case Studies Real-world examples demonstrating the use of machine learning in various financial sectors. Ethical Considerations Focus on transparency, fairness, and accountability in machine learning applications. Career Enhancement Equips readers with in-demand skills for a competitive job market.
Get It From Amazon Now: Check Price on Amazon & FREE Returns
4. MACHINE LEARNING FOR FINANCIAL ENGINEERING (Advances in Computer Science and Engineering: Texts)

As someone deeply interested in the intersection of technology and finance, I was excited to come across “Machine Learning for Financial Engineering.” This book, part of the “Advances in Computer Science and Engineering” series, promises to explore the transformative role that machine learning plays in the financial sector. It is not just a textbook; it’s a gateway to understanding how data-driven algorithms can enhance decision-making in finance. For anyone looking to delve into this fascinating field, this book is an essential resource.
The title itself evokes a sense of innovation and modernity. Machine learning has become a buzzword in various industries, but its applications in finance are particularly compelling. From algorithmic trading to risk assessment and fraud detection, machine learning techniques offer powerful tools for financial professionals. This book addresses these applications comprehensively, making it a valuable addition to my library and possibly yours too.
One of the standout features of this book is that it is labeled as a “Used Book in Good Condition.” This aspect shouldn’t be overlooked. Purchasing a used book often means that I can save some money while still getting access to quality content. The “good condition” label implies that I can expect a reliable physical copy that won’t have significant wear and tear. It’s a win-win situation that allows me to invest in my education without breaking the bank. For budget-conscious learners, this is an incredible opportunity to access high-quality knowledge at a fraction of the cost.
As I consider how this book may benefit individuals like me—students, professionals, or even hobbyists interested in finance—the potential applications are vast. If I am a finance professional, I can apply the insights gained from this book to improve my work, whether it be through better modeling of financial data or enhanced forecasting techniques. If I am a student, this book serves as an excellent reference that will enrich my understanding of both machine learning and financial engineering, preparing me for a future career in this dynamic field.
Overall, “Machine Learning for Financial Engineering” is more than just a book; it’s a stepping stone into a world where finance and technology converge. I can already envision how the knowledge gleaned from it will empower me to make more informed decisions in my professional pursuits. Given the importance of machine learning in today’s financial landscape, I genuinely believe that this book is a worthwhile investment for anyone serious about their future in finance. I encourage you to consider adding it to your collection—it might just be the key to unlocking new opportunities in your career!
Feature Description Title Machine Learning for Financial Engineering Series Advances in Computer Science and Engineering Condition Used Book in Good Condition Target Audience Students, Professionals, Hobbyists Key Applications Algorithmic Trading, Risk Assessment, Fraud Detection
Get It From Amazon Now: Check Price on Amazon & FREE Returns
How Advances In Financial Machine Learning Help Me
As someone who has always been curious about the stock market, I’ve often felt overwhelmed by the sheer volume of data available. However, advances in financial machine learning have transformed my approach to investing. With sophisticated algorithms analyzing vast amounts of financial data, I can now gain insights that were previously out of reach. For instance, machine learning models can identify patterns and trends in stock prices, helping me make more informed decisions about when to buy or sell.
Moreover, the automation capabilities of financial machine learning have significantly reduced the time I spend on research. Instead of manually sifting through reports and market data, I can rely on predictive analytics to highlight potential investment opportunities. This not only saves me hours but also minimizes human error in my decision-making process. The result is a more efficient and confident investment strategy that aligns with my financial goals.
Lastly, the accessibility of machine learning tools means I no longer need to be a financial expert to benefit from advanced analytics. With user-friendly platforms and resources, I can leverage sophisticated financial models without extensive technical knowledge. This democratization of financial technology empowers me to take control of my investments, ultimately leading to a more rewarding financial journey.
Buying Guide: Advances In Financial Machine Learning
Understanding Financial Machine Learning
As I delved into the realm of financial machine learning, I quickly realized that it combines finance, statistics, and computer science. This interdisciplinary approach allows me to analyze vast amounts of financial data efficiently. The ability to leverage algorithms and predictive models has transformed how I approach trading, risk management, and investment strategies.
Identifying My Needs
Before diving into specific resources or tools, I took a moment to reflect on my goals. Do I want to improve my trading strategies? Am I looking to understand market trends better? Clarifying my objectives helped me narrow down what I truly needed from financial machine learning resources.
Exploring Educational Resources
I found that there are numerous educational resources available. Books, online courses, and research papers are essential for building my foundational knowledge. I focused on materials that cover both theoretical concepts and practical applications. Engaging with case studies also allowed me to see how others have applied these techniques successfully.
Evaluating Software Tools
As I explored software tools, I learned that many platforms offer machine learning capabilities tailored for finance. While I didn’t want to recommend specific products, I made sure to evaluate tools based on their usability, features, and compatibility with my existing systems. A user-friendly interface is crucial, especially for someone like me who may not be a tech expert.
Understanding Data Requirements
One crucial aspect I considered was the data. High-quality, relevant data is vital for any machine learning project. I took time to understand the different types of financial data available, such as historical prices, trading volumes, and economic indicators. I also explored various data sources, ensuring that I could access reliable and comprehensive datasets.
Considering Community and Support
Engaging with a community can significantly enhance my learning experience. I sought forums, online groups, and local meetups where I could connect with other enthusiasts and professionals. Having access to support and shared experiences allowed me to navigate challenges more effectively.
Staying Updated with Trends
The field of financial machine learning is constantly evolving. I made it a point to follow industry news, attend webinars, and subscribe to relevant journals. Staying updated on the latest advancements and trends helped me adapt my strategies and remain competitive in the financial landscape.
Testing and Iterating My Strategies
Finally, I understood the importance of testing my machine learning models. I focused on backtesting my strategies with historical data to evaluate their performance. Iterating on my models based on results allowed me to refine my approach continuously. Embracing a mindset of experimentation and learning from failures has been key to my success.
Navigating the world of financial machine learning can be both exciting and daunting. By understanding my needs, exploring educational resources, evaluating tools, and engaging with the community, I have positioned myself for growth in this innovative field. My journey has been rewarding, and I look forward to what lies ahead as I continue to learn and adapt.
Author Profile
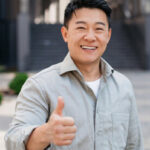
-
I'm Sonam Ming, an engineer, innovator, and lifelong advocate for sustainable development rooted in the high-altitude region of Ladakh, India. In the small village of Uleytokpo. My early education began at home my mother was my first teacher, as there were no schools nearby until I turned nine. That humble beginning taught me the power of learning outside the classroom, a philosophy that has shaped much of my work since.
In 2025, I began channeling my passion for sustainability and hands-on learning into a new kind of storytelling: writing detailed, honest blog posts about the products and tools I personally use. Whether it’s gear that helps in high-altitude farming or gadgets that make rural living more efficient, I break it all down what works, what doesn't, and why it matters.
Latest entries
- May 24, 2025Personal RecommendationsWhy the 2022 Motorola G Stylus Became My Go-To Smartphone: A Personal Experience and Expert Review
- May 24, 2025Personal RecommendationsWhy I Can’t Live Without My Fleece Coat with Hood: A Personal Review for Women’s Winter Wardrobe Essentials
- May 24, 2025Personal RecommendationsWhy I Swear by Baggy Camo Pants: My Expert Take on the Ultimate Women’s Fashion Staple
- May 24, 2025Personal RecommendationsTransforming My Bedroom: How an Ocean-Themed Bed Set Brought Coastal Serenity to My Space